Application of machine learning in the prediction of college students' suicidal ideation
-
摘要:
目的 探索机器学习算法在预测大学生是否存在自杀意念中的效果, 并分析大学生自杀意念的危险因素。 方法 选取某高校2021年21 224名在校本科生心理数据。以37项人口学和内外在心理因素为自变量, 以大学生是否存在自杀意念为因变量, 使用支持向量机、随机森林和LightGBM算法分别建立预测模型。将模型应用于测试集上, 以检出率、F1分数和准确率评价预测效果。基于较优模型分析大学生自杀意念的高风险因素。 结果 支持向量机、随机森林和LightGBM模型的检出率依次为0.61, 0.64, 0.69;F1分数依次为0.63, 0.63, 0.64;准确率依次为0.73, 0.73, 0.72。基于较优的LightGBM模型分析大学生自杀意念高风险因素, 按照重要性排序依次为抑郁、年级、性别、绝望、生源地、拥有意义感、对自杀的态度、依赖、家庭经济情况、幻觉妄想症状、焦虑、网络成瘾和人际关系困扰。 结论 LightGBM模型预测大学生是否存在自杀意念相较于支持向量机和随机森林模型有较好的预测效果。 Abstract:Objective To explore the predictive effect of machine learning algorithms on college students'suicidal ideation and to analyze the associated factors of college students'suicidal ideation. Methods The mental health data of 21 224 undergraduates was selected from a university in 2021.The independent variables were 37 demographic and internal and external mental health factors.The dependent variable was whether college students had suicidal ideation.Support vector machine, random forest and LightGBM algorithm were used to establish prediction models.The model was used in test set to so as to evaluate the model's prediction effect by using detection rate, F1 score and accuracy rate.Based on the superior model, the high-risk factors of suicidal ideation in college students were analyzed. Results The detection rates of support vector machine, random forest, and LightGBM models were 61.0%, 64.0%, 69.0%;F1 scores were 0.63, 0.63, 0.64, and accuracy rates were 73.0%, 73.0%, 72.0%, respectively.Based on the superior LightGBM model, risk factors of suicidal ideation in college students included, depression, grade, gender, despair, place of origin, sense of meaning, attitude toward suicide, dependence, family economic situation, hallucinatory delusion symptoms, anxiety, internet addiction, and interpersonal distress. Conclusion The LightGBM model has a better prediction effect than the support vector machine and random forest models. -
Key words:
- Suicide /
- Consciousness /
- Mental health /
- Models, statistical /
- Students
1) 利益冲突声明 所有作者声明无利益冲突。2) 王苗苗 -
表 1 大学生一般情况在有无自杀意念组间分布比较
Table 1. Comparison of basic information of college students between groups with or without suicidal ideation
组别 无自杀意念(n=13 464) 有自杀意念(n=7 760) χ2值 P值 性别 男 7 222(53.64) 3 589(46.25) 107.56 < 0.01 女 6 242(46.36) 4 171(53.75) 年级 大一 3 735(27.74) 2 597(33.47) 83.05 < 0.01 大二 3 471(25.78) 1 875(24.16) 大三 3 028(22.49) 1 523(19.63) 大四 2 879(21.38) 1 551(19.99) 大五及以上 351(2.61) 214(2.76) 民族 汉族 12 873(95.61) 7 405(95.43) 0.40 0.53 少数民族 591(4.39) 355(4.57) 生源地 农村 6 444(47.86) 3 560(45.88) 8.75 0.01 城镇 3 856(28.64) 2 347(30.24) 城市 3 164(23.50) 1 853(23.88) 留守经历 有 3 354(24.91) 2 305(29.70) 57.83 < 0.01 无 10 110(75.09) 5 455(70.30) 对学校专业满意情况 满意 11 039(81.99) 5 454(70.28) 389.40 < 0.01 不满意 2 425(18.01) 2 306(29.72) 家庭经济情况 非常贫困 279(2.07) 174(2.24) 12.46 0.01 比较贫困 2 582(19.18) 1 609(20.73) 一般 9 063(67.31) 5 175(66.69) 较好 1 498(11.13) 779(10.04) 非常好 42(0.31) 23(0.30) 每月生活费/元 < 600 85(0.63) 64(0.82) 4.96 0.29 600~ < 1 000 2 006(14.90) 1 126(14.51) 1 000~ < 1 500 6 698(49.75) 3 812(49.12) 1 500~ < 2 000 3 584(26.62) 2 134(27.50) ≥2 000 1 091(8.10) 624(8.04) 家庭结构 完整家庭 12 583(93.46) 7 054(90.90) 46.43 < 0.01 单亲、失亲家庭 881(6.54) 706(9.10) 父母婚姻状况 良好 10 369(77.01) 4 806(61.93) 568.71 < 0.01 一般 2 027(15.05) 1 903(24.52) 离异 716(5.32) 613(7.90) 较差、分居 352(2.61) 438(5.64) 严重的慢性疾病或身体残疾 有 119(0.88) 150(1.93) 43.30 < 0.01 无 13 345(99.12) 7 610(98.07) 亲友有自杀及自杀未遂行为 有 466(3.46) 707(9.11) 300.94 < 0.01 无 12 998(96.54) 7 053(90.89) 对自杀的态度 排斥 12 484(92.72) 4 755(61.28) 3 195.31 < 0.01 无所谓 801(5.95) 2 363(30.45) 接受 179(1.33) 642(8.27) 注:()内数字为构成比/%。 表 2 有无自杀意念大学生心理特征得分比较/[M(P25, P75)]
Table 2. Comparison of mental characteristics scores of college students with or without suicidal ideation/[M(P25, P75)]
有无自杀意念 人数 绝望 幻觉妄想症状 焦虑 抑郁 偏执 自卑 无 7 760 3.00(2.00, 5.00) 1.00(1.00, 1.25) 1.50(1.00, 2.00) 1.40(1.20, 2.00) 1.50(1.00, 2.00) 1.60(1.20, 2.00) 有 13 464 6.00(4.00, 9.00) 1.25(1.00, 1.75) 2.00(1.50, 2.50) 2.00(1.60, 2.40) 1.75(1.25, 2.25) 2.00(1.60, 2.40) H值 53.87 34.06 46.50 52.88 39.23 46.89 有无自杀意念 人数 敏感 社交恐惧 躯体化 依赖 敌对攻击 冲动 无 7 760 1.75(1.50, 2.25) 1.75(1.25, 2.00) 1.00(1.00, 1.50) 1.75(1.25, 2.00) 1.25(1.00, 1.75) 1.75(1.25, 2.00) 有 13 464 2.25(1.75, 2.75) 2.00(1.50, 2.50) 1.25(1.00, 2.00) 2.00(1.50, 2.25) 1.50(1.25, 2.00) 2.00(1.75, 2.50) H值 42.46 37.14 36.09 30.79 36.40 40.03 有无自杀意念 人数 强迫 网络成瘾 自伤行为 进食问题 睡眠困扰 学校适应困难 无 7 760 1.75(1.25, 2.25) 2.00(1.40, 2.40) 1.00(1.00, 1.25) 1.25(1.00, 1.50) 1.75(1.25, 2.00) 1.75(1.50, 2.00) 有 13 464 2.25(1.75, 2.50) 2.20(1.80, 2.80) 1.00(1.00, 1.50) 1.50(1.25, 1.75) 2.00(1.50, 2.50) 2.00(1.75, 2.50) H值 39.78 25.69 31.03 28.20 37.57 35.66 有无自杀意念 人数 人际关系困扰 学业压力 就业问题 恋爱困扰 拥有意义感 寻求意义感 无 7 760 1.75(1.25, 2.00) 2.25(2.00, 2.75) 2.25(2.00, 3.00) 1.50(1.00, 2.00) 26.00(23.00, 30.00) 27.00(24.00, 30.00) 有 13 464 2.00(1.50, 2.25) 2.50(2.25, 3.00) 2.75(2.25, 3.00) 1.75(1.25, 2.00) 23.00(19.00, 27.00) 27.00(24.00, 30.00) H值 37.76 32.01 36.85 20.32 -42.40 -5.84 注:P值均 < 0.01;“绝望”由贝克绝望量表测得,“拥有意义感”和“寻求意义感”由生命意义感量表测得,其余指标由中国大学生心理健康筛查量表测得。 表 3 不同机器学习算法预测大学生自杀意念效果及参数情况
Table 3. Effects and parameters of different machine learning algorithms
算法 检出率/% F1分数 准确率/% 参数 支持向量机 61.0 0.63 73.0 核函数:RBF,惩罚系数:1 随机森林 64.0 0.63 73.0 学习器数量:50,单个学习器选择的最大特征数目:8,结点最小分裂样本数:80,叶子结点最小样本数:20,决策树最大深度:10,使用袋外样本 LightGBM 69.0 0.64 72.0 学习器数量:4,每棵决策树叶子数量:4,叶子结点最小样本数:12,学习率:1,L1正则化项:0.000 977,L2正则化惩罚系数:1 024 -
[1] 陆卓林, 梁瑞琼, 邱鸿钟, 等. 南方某省高校大学生2013-2018年自杀现状[J]. 中国学校卫生, 2019, 40(7) : 1085-1087. doi: 10.16835/j.cnki.1000-9817.2019.07.035LU Z L, LIANG R Q, QIU H Z, et al. Suicide status of college students in a province in South China during 2013-2018[J]. Chin J Sch Health, 2019, 40(7) : 1085-1087. doi: 10.16835/j.cnki.1000-9817.2019.07.035 [2] 杨振斌, 李焰. 大学生非正常死亡现象的分析[J]. 心理与行为研究, 2015, 13(5) : 698-701. doi: 10.3969/j.issn.1672-0628.2015.05.017YANG Z B, LI Y. An analysis on unnatural deaths of college students[J]. Studi Psychol Behav, 2015, 13(5) : 698-701. doi: 10.3969/j.issn.1672-0628.2015.05.017 [3] KLONSKY E D, MAY A M, SAFFER B Y. Suicide, suicide attempts, and suicidal ideation[J]. Ann Rev Clin Psychol, 2016, 12(1) : 307-330. doi: 10.1146/annurev-clinpsy-021815-093204 [4] BECK A T, STEER R A, RANIERI W F. Scale for suicide ideation: psychometric properties of a self-report version[J]. J Clin Psychol, 1988, 44(4) : 499-505. doi: 10.1002/1097-4679(198807)44:4<499::AID-JCLP2270440404>3.0.CO;2-6 [5] MIRANDA R, ORTIN A, SCOTT M, et al. Characteristics of suicidal ideation that predict the transition to future suicide attempts in adolescents[J]. J Child Psychol Psychiatry, 2014, 55(11) : 1288-1296. doi: 10.1111/jcpp.12245 [6] KLONSKY E D, MAY A M. The Three-Step Theory (3ST): a new theory of suicide rooted in the "ideation-to-action" framework[J]. Int J Cogn Ther, 2015, 8(2) : 114-129. doi: 10.1521/ijct.2015.8.2.114 [7] MICHé M, STUDERUS E, MEYER A H, et al. Prospective prediction of suicide attempts in community adolescents and young adults, using regression methods and machine learning[J]. J Affect Disord, 2020, 265: 570-578. doi: 10.1016/j.jad.2019.11.093 [8] BHAK Y, JEONG H, CHO Y S, et al. Depression and suicide risk prediction models using blood-derived multi-omics data[J]. Transl Psychiatr, 2019, 9(1) : 262. doi: 10.1038/s41398-019-0595-2 [9] RYU S, LEE H, LEE D K, et al. Detection of suicide attempters among suicide ideators using machine learning[J]. Psychiatry Investig, 2019, 16(8) : 588-593. doi: 10.30773/pi.2019.06.19 [10] BARROS J, MORALES S, ECHáVARRI O, et al. Suicide detection in Chile: proposing a predictive model for suicide risk in a clinical sample of patients with mood disorders[J]. Rev Bras Psiquiatr, 2016, 39(1) : 1-11. doi: 10.1590/1516-4446-2015-1877 [11] METZGER M H, TVARDIK N, GICQUEL Q, et al. Use of emergency department electronic medical records for automated epidemiological surveillance of suicide attempts: a French pilot study[J]. Int J Methods Psychiatr Res, 2017, 26(2) : e1522. doi: 10.1002/mpr.1522 [12] ROZENBAUM D, SHREVE J, RADAKOVICH N, et al. Personalized prediction of hospital mortality in COVID-19 positive patients[J]. Mayo Clin Proc Inn Qual Out, 2021, 5(4) : 795-801. doi: 10.1016/j.mayocpiqo.2021.05.001 [13] 欧阳平, 李小溪, 冷芬, 等. 机器学习算法在体检人群糖尿病风险预测中的应用[J]. 中华疾病控制杂志, 2021, 25(7) : 849-853, 868. https://www.cnki.com.cn/Article/CJFDTOTAL-JBKZ202107020.htmOUYANG P, LI X X, LENG F, et al. Application of machine learning algorithm in diabetes risk prediction of physical examination population[J]. Chin J Dis Control Prev, 2021, 25(7) : 849-853, 868. https://www.cnki.com.cn/Article/CJFDTOTAL-JBKZ202107020.htm [14] 方晓义, 袁晓娇, 胡伟, 等. 中国大学生心理健康筛查量表的编制[J]. 心理与行为研究, 2018, 16(1): 111-118. doi: 10.3969/j.issn.1672-0628.2018.01.015FANG X Y, YUAN X J, HU W, et al. The development of college students mental health screening Scale[J]. Stud Psychol Behav, 2018, 16(1): 111-118. doi: 10.3969/j.issn.1672-0628.2018.01.015 [15] 孔媛媛. Beck绝望量表中文版在青少年中使用的信度和效度[J]. 中国心理卫生杂志, 2007, 21(10): 686-689. doi: 10.3321/j.issn:1000-6729.2007.10.008KONG Y Y. Reliability and validity of the Beck Hopelessness Scale for adolescent[J]. Chin Ment Health J, 2007, 21(10): 686-689. doi: 10.3321/j.issn:1000-6729.2007.10.008 [16] 刘思斯, 甘怡群. 生命意义感量表中文版在大学生群体中的信效度[J]. 中国心理卫生杂志, 2010, 24(6): 478-482. https://www.cnki.com.cn/Article/CJFDTOTAL-ZXWS201006029.htmLIU S S, GAN Y Q. Reliability and validity of the Chinese version of the meaning in life questionnaire[J]. Chin Ment Health J, 2010, 24(6): 478-482. https://www.cnki.com.cn/Article/CJFDTOTAL-ZXWS201006029.htm [17] 李献云, 费立鹏, 张亚利, 等. Beck自杀意念量表中文版在大学学生中应用的信效度[J]. 中国心理卫生杂志, 2011, 25(11): 862-866. doi: 10.3969/j.issn.1000-6729.2011.11.013LI X Y, FEI L P, ZHANG Y L, et al. Reliability and validity of the Chinese version of Beck Scale for suicide ideation (BSI-CV) among university students[J]. Chin Ment Health J, 2011, 25(11) : 862-866. doi: 10.3969/j.issn.1000-6729.2011.11.013 [18] 寇毛蕊, 冯志远, 杨新国. 大学生自杀意念影响因素Meta分析[J]. 中国预防医学杂志, 2018, 19(7) : 520-526. https://www.cnki.com.cn/Article/CJFDTOTAL-ZGYC201807009.htmKOU M R, FENG Z Y, YANG X G. Influencing factors of suicidal ideation of college students: a Meta-analysis[J]. Chin Prev Med, 2018, 19(7) : 520-526. https://www.cnki.com.cn/Article/CJFDTOTAL-ZGYC201807009.htm [19] 王沫涵. 吉林省在校大学生自杀意念及其影响因素分析[D]. 长春: 吉林大学, 2019.WANG M H. Study on suicidal ideation and its influencing factors among college students in Jilin Province[D]. Changchun: Jilin University, 2019. [20] 巢传宣. 我国大学生自杀意念的影响因素[J]. 保健医学研究与实践, 2018, 15(6) : 81-85, 90. https://www.cnki.com.cn/Article/CJFDTOTAL-GXBJ201806025.htmCHAO C X. Influence factors of suicidal ideation among college students in China[J]. Health Med Res Pract, 2018, 15(6) : 81-85, 90. https://www.cnki.com.cn/Article/CJFDTOTAL-GXBJ201806025.htm [21] 高世伟, 柳晓琳. 吉林市大学生生命质量与自杀意念相关性及自杀意念的影响因素分析[J]. 现代预防医学, 2020, 47(10) : 1848-1851. https://www.cnki.com.cn/Article/CJFDTOTAL-XDYF202010031.htmGAO S W, LIU X L. Quality of life correlated with suicidal ideation and the influencing factors of suicidal ideation analysis among undergraduate students in Jilin city[J]. Mod Prev Med, 2020, 47(10) : 1848-1851. https://www.cnki.com.cn/Article/CJFDTOTAL-XDYF202010031.htm [22] 陈君, 耿仁文. 心理健康与自杀行为的影响因素: 基于对三所高校10340名医学生的调查[J]. 南方医科大学学报, 2020, 40(11) : 1689-1693. doi: 10.12122/j.issn.1673-4254.2020.11.24CHEN J, GENG R W. Factors affecting psychological health and suicidal behavior: based on a survey of 10 340 medical students from three universities[J]. J South Med Univ, 2020, 40(11) : 1689-1693. doi: 10.12122/j.issn.1673-4254.2020.11.24 [23] 李旭, 郑涵予, 卢勤. 大学生自杀意念及其影响因素分析[J]. 中国公共卫生, 2016, 32(3) : 359-362. https://www.cnki.com.cn/Article/CJFDTOTAL-ZGGW201603029.htmLI X, ZHENG H Y, LU Q. Suicidal ideation and associated factors among university students[J]. Chin J Public Health, 2016, 32(3) : 359-362. https://www.cnki.com.cn/Article/CJFDTOTAL-ZGGW201603029.htm [24] 黄彦. 青少年网络成瘾者自杀行为特征及自杀风险评估[D]. 重庆: 重庆医科大学, 2018.HUANG Y. Suicidal behavior characteristics and suicide risk assessment of adolescent with internet addiction[D]. Chongqing: Chongqing Medical University, 2018. [25] TEZCAN J, CHENG Q. Support vector regression for estimating earthquake response spectra[J]. Bull Earthq Eng, 2012, 10(4) : 1205-1219. doi: 10.1007/s10518-012-9350-2 [26] 梁子超, 李智炜, 赖铿, 等. 10折交叉验证用于预测模型泛化能力评价及其R软件实现[J]. 中国医院统计, 2020, 27(4) : 289-292. doi: 10.3969/j.issn.1006-5253.2020.04.001LIANG Z C, LI Z W, LAI K, et al. Application of 10-fold cross-validation in the evaluation of generalization ability of prediction models and the realization in R[J]. Chin J Hospit Statis, 2020, 27(4) : 289-292. doi: 10.3969/j.issn.1006-5253.2020.04.001 [27] 石洪波, 陈雨文, 陈鑫. SMOTE过采样及其改进算法研究综述[J]. 智能系统学报, 2019, 14(6) : 1073-1083. https://www.cnki.com.cn/Article/CJFDTOTAL-ZNXT201906002.htmSHI H B, CHEN Y W, CHEN X. Summary of research on SMOTE oversampling and its improved algorithms[J]. CAAI Transact Intell Systems, 2019, 14(6) : 1073-1083. https://www.cnki.com.cn/Article/CJFDTOTAL-ZNXT201906002.htm [28] POWERS D M W. Evaluation: from precision, recall and f-measure to ROC, informedness, markedness and correlation[J]. J Mach Learn Technol, 2011, 2(1) : 37-63. [29] 李磊, 黄水平. 支持向量机原理及其在医学分类中的应用[J]. 中国卫生统计, 2009, 26(1) : 22-25. doi: 10.3969/j.issn.1002-3674.2009.01.006LI L, HUANG S P. The principle of support vector machine and its application in medical classification[J]. Chin J Heal Stat, 2009, 26(1) : 22-25. doi: 10.3969/j.issn.1002-3674.2009.01.006 [30] BREIMAN L. Random forests[J]. Mach Learn, 2001, 45(1) : 5-32. doi: 10.1023/A:1010933404324 [31] KE G, MENG Q, FINLEY T, et al. LightGBM: a highly efficient gradient boosting decision tree[J]. Adv Neural Inf Process Syst, 2017, 30: 3146-3154. [32] 王芳杰, 王福建, 王雨晨, 等. 基于LightGBM算法的公交行程时间预测[J]. 交通运输系统工程与信息, 2019, 19(2) : 116-121. https://www.cnki.com.cn/Article/CJFDTOTAL-YSXT201902017.htmWANG F J, WANG F J, WANG Y C, et al. Bus travel time prediction based on light gradient boosting machine algorithm[J]. J Transport Systems Engin Inform Technol, 2019, 19(2) : 116-121. https://www.cnki.com.cn/Article/CJFDTOTAL-YSXT201902017.htm [33] SHUAI Y, ZHENG Y, HUANG H. Hybrid software obsolescence evaluation model based on PCA-SVM-GridSearchCV[C]//2018 IEEE 9th international conference on software engineering and service science (ICSESS) IEEE. Beijing: IEEE, 2018: 449-453. -
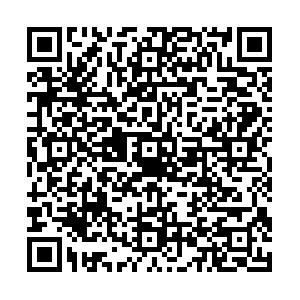
计量
- 文章访问数: 917
- HTML全文浏览量: 303
- PDF下载量: 136
- 被引次数: 0